DigitalTwin-4-Multiphysics-Lab
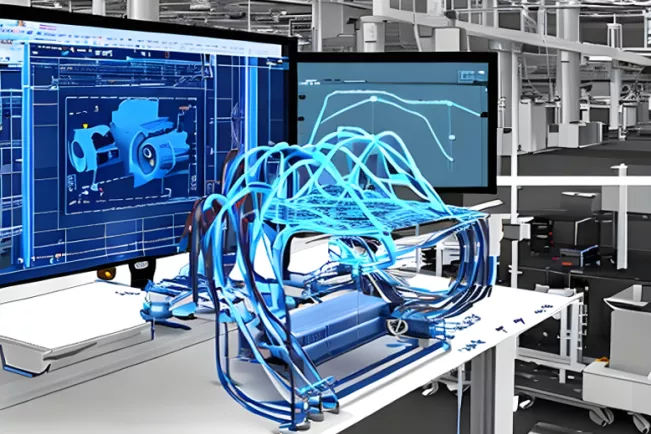
Research project at a glance
Period
01.04.2023 to 30.06.2030
Project Description
The "Digital Twin-4-Multiphysics Lab" (DT4MP) is equally dedicated to the topics of urban digital twins (UDTs) and multiphysics twins for industry. Both areas are addressed on an equal footing and in synergy to develop innovative solutions and optimizations for urban and industrial applications.
Multiphysics phenomena are of central importance in both urban digital twins (UDTs) and many industrial applications and production lines. These phenomena influence the efficiency and safety of processes as well as the integrity of the produced parts. Embedded in process chains and design tasks, multiphysics simulations are subject to significant automation and optimization for both urban and industrial applications. These simulations are an integral part of digital twins – virtual, functional versions of systems that capture their properties and represent the lifecycle of a system in the form of data and metadata.
In the area of urban digital twins (UDTs), DT4MP enables detailed analysis and optimization of urban processes and infrastructures. At the same time, multiphysics twins in industry contribute to increased efficiency and process safety. Through the integration of real-time data and advanced simulation models, UDTs contribute to improving the quality of life, efficient resource use, and promoting sustainable urban development. In industry, similar technologies are used to optimize production and ensure the quality of produced parts.
DT4MP combines digital twins and artificial intelligence (AI) for both urban and industrial applications. New methods are developed and applied through the combination of machine learning and multiphysics simulations. The lab offers services such as data analysis, virtual testing, and validation, benefiting both small and medium-sized enterprises (SMEs) and larger companies in urban and industrial contexts. It promotes cooperation in research and organizes regular discussions around digital twins.
Fraunhofer SCAI, the Institute for Technology, Resource Conservation and Energy Efficiency (TREE), the Institute for Artificial Intelligence and Autonomous Systems (A2S) at the Bonn-Rhein-Sieg University of Applied Sciences, and the Dr. Reinold Hagen Foundation founded DT4MP to advance digitalization research in industry and urban areas and to support SMEs.
Results
Current projects, initiatives and results
EcoTwin – Digital Twin of Urban Green Spaces
PI/Institute: Tim Wessel, Prof. Dr. Martin Hamer (IZNE)
How can a digital twin of urban green spaces be designed to enable regional environmental and green space offices to practically implement climate change adaptation measures based on specific site characteristics?
Data: Environmental data (open source, provided by cities, sensor data)
Technology and Methodology: Sensor technology, Natural Language Processing, API (weather and sensor data)
Partners: RF-Frontend GmbH, TH Köln, GIQS e.V.
Garrulus-2
PI/Institute: Prof Dr. Alexander Asteroth (TREE), Prof. Dr. Sebastian Houben (A2S/TREE)
How can forest management be economically conducted using drone-supported multi-modal sensor technology to create a digital twin of a forest area?
Data: Image data (including hyperspectral), 3D point clouds, satellite data of forest areas
Technology and Methodology: Autonomous robotics, simultaneous localization and mapping, multi-sensor integration, data-driven machine learning, few-shot learning, continual learning
Partners: Wald und Holz NRW
OpenSKIZZE: Open Source Development Tools for Urban Planning: Climate Adaptation with Cooperative AI-Supported Decision-Making Processes
PI/Institute: Dr. Alexander Hagg (TREE), Prof. Dr. Dirk Reith (TREE)
OpenSKIZZE is intended to be an open-source AI assistant that translates insights from climate models into specific construction projects. This system aims to involve all stakeholders early in the process and educate them on the impacts of their decisions on the local urban climate.
Data: Geodata, climate data
Technology and Methodology: Machine learning, evolutionary optimization, neural representation methods, generative AI, computational fluid dynamics
Partners: Montag Stiftung Urbane Räume gAG, Neue Stadtgärtnerei Wohnen e.V.
Data Competence Center Rhein-Ruhr
PI/Institute: Prof. Dr. Dirk Reith (TREE), Prof. Dr. Sebastian Houben (A2S/TREE), Dr. Armin Erhardt
Offering the integration of natural and humanities research with modern data-driven methods and best practices.
Data: Large-scale natural science measurement data
Technology and Methodology: Dependent on the supported project
Partners: FZ Jülich GmbH (Consortium Coordination), RWTH Aachen, FH Aachen, Universität zu Köln, Rheinische Friedrich-Wilhelms-Universität Bonn, Fraunhofer - Institut für Intelligente Analyse- und Informationssysteme, Universität Duisburg-Essen, Ruhr-Universität Bochum
KIMODE - AI Methodology for Optimizing Design-of-Experiments
PI/Institute: Prof. Dr. Sebastian Houben (A2S/TREE), Prof. Dr. Dirk Reith (TREE)
Can components within a design-of-experiments framework be developed faster and more accurately using AI-based methodologies?
Data: CAD designs, simulation data on durability/CO2 footprint
Technology and Methodology: Physics-informed deep learning, Bayesian optimization, e.g., Gaussian processes
Partners: GKN Driveline
NAkSU – New Analytical Methods for Complex Safety and Environmental Data
PI/Institute: Prof. Dr. André Hinkenjann (IVC), Prof. Dr. Ernst Kruijff (IVC), Prof. Dr. Peter Kaul (ISF), Prof. Dr. Robert lange (ISF)
Advanced research on visualizing complex sensor data and improving data quality from simple, cost-effective sensors.
Data: Environmental data measured with mobile and stationary multi-sensor systems (temperature, humidity, air pollutants such as ozone, nitrogen oxides, ozone, fine particles), GPS data; third-party measurement data
Technology and Methodology: Cost-effective sensors, e.g., electrochemical cells, optical fine dust sensor, photoionization detector, quality assurance methods, open-source development tools; visual preparation of results for a common language among all participants
Partners: Stadt Lohmar
https://www.h-brs.de/en/naksu-new-analysis-methods-complex-safety-and-environmental-data
KLUGER Transfer (Climate-Environment-Health Transfer), Knowledge Transfer in the Areas of Climate, Environment, and Health
PI/Institute: Prof. Dr. Wiltrud Terlau, Angela Turck (IZNE)
Increasing knowledge transfer from basic research at the Max Planck Institute for Chemistry through applied research at the International Center for Sustainable Development of the Bonn-Rhein-Sieg University of Applied Sciences to the public, politics, and economy.
Data: Qualitative and quantitative data collection
Methodology: Interviews, surveys, focus groups (Mixed Method Approach)
Partners: Max-Planck-Institut für Chemie (MPIC), Kommunale Politik und Verwaltung, regionale Wirtschaft