Department of Computer Science
Open source machine learning in computational chemistry
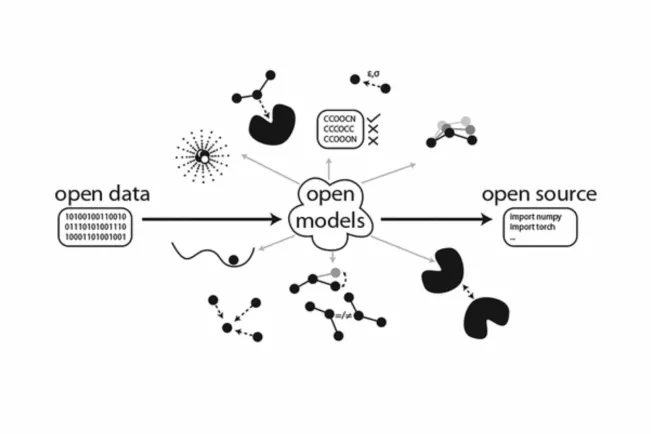
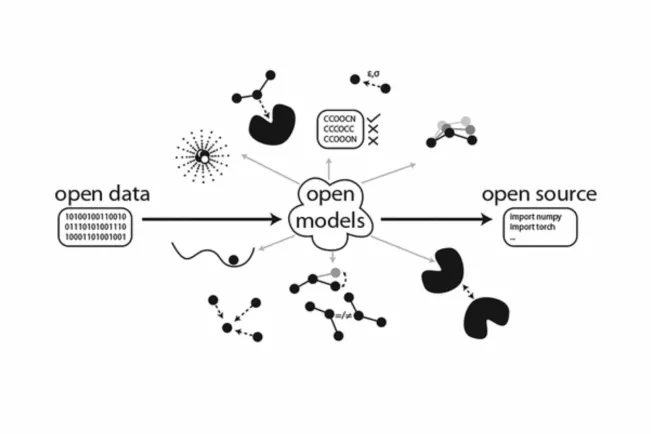
In the field of computational chemistry, the integration of machine learning concepts and algorithms has increased significantly. To this end, Hagg and Kirschner examined 179 open source software projects, through peer-reviewed articles, to gain a better understanding of the molecular topics being investigated using machine learning approaches.
For each project, Hagg and Kirschner provide a short description, a link to the code, the associated licence type and whether the training data and the resulting models are publicly available. Based on the projects deposited GitHub repositories, the most frequently used Python libraries are indentified. This overview will serve as a resource to learn more about how machine learning can be applied to computational chemistry topics by identifying accessible codes with accompanying papers on a topic-by-topic basis. To this end, open source computational chemistry software for generating training data and basic Python machine learning libraries were also included. Based on the observations and considering the three pillars of collaborative work in machine learning - open data, open sources code and open models - the researchers make some suggestions to the community.
Kontakt

Alexander Hagg
PhD student, Artificial intelligence (surrogate modelling), Artificial Intelligence (Optimisation)
Research fields
Location
Sankt Augustin
Address
Grantham-Allee 20
53757 Sankt Augustin

Karl Kirschner
Research Scientist, Mentor and Teacher, International Chair (2017-'19)
Research fields
Location
Sankt Augustin
Room
C 228
Address
Grantham-Allee 20
53754, Sankt Augustin
Telephone
+49 2241 865 267