Fachbereich Informatik
Authorship on the Dark Web: Best Paper Award for Alumna
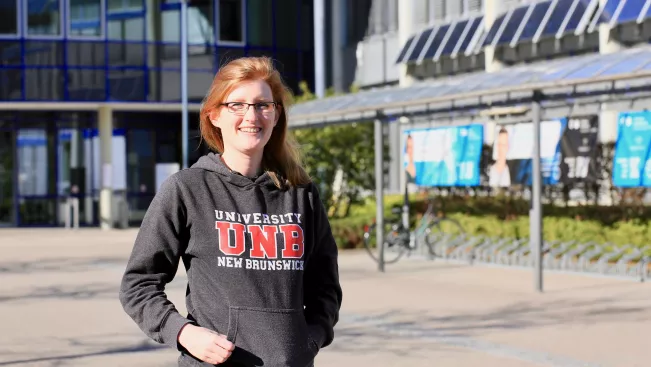
Britta Sennewald is a graduate of the Dual Degree Programme of Hochschule Bonn-Rhein-Sieg and the Canadian partner institution University of New Brunswick (UNB). She studied computer science at H-BRS and chose to focus on cyber security, especially cryptography, in her Master's degree. Her Master's thesis dealt with the Dark Web and was awarded the Best Thesis Award of the University Society in 2020. She then continued her research at UNB in Canada. As a graduate of the Dual Degree Programme, she earned two Master's degrees, one in Germany and one at UNB.
Now she published her first paper "Voting for Authorship Attribution Applied to Dark Web Data" together with her supervisor Prof. Dr. Kenneth Kent (UNB, Honorary Professor at H-BRS), Prof. Dr. Rainer Herpers and Dr. Marco Hülsmann (both H-BRS).
Sennewald and her German-Canadian research team were awarded the Best Paper Award of the IBM Centre for Advanced Studies in Computer Science for their work at the 30th Annual International Conference on Computer Science and Software Engineering (CASCON'20).
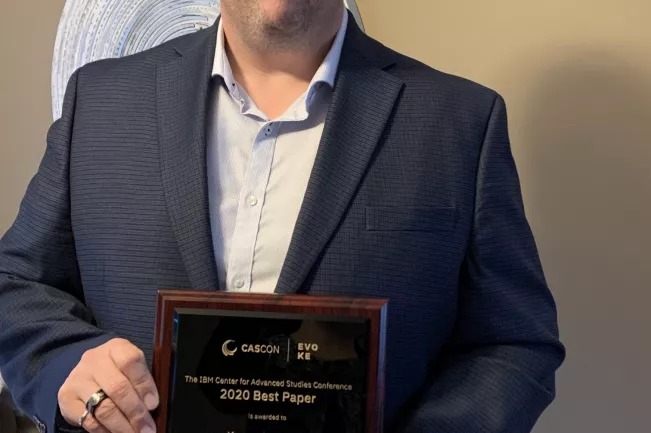
Research topic: Authorship on the Dark Web
The part of the Internet that is not indexed or searchable by normal search engines is called the Deep Web. Within the Deep Web, the Dark Web is a less accessible subset of areas that require special software, tools or connections to access. In recent years, the Dark Web has gained popularity in certain communities because it offers a higher level of privacy and anonymity. Like the Surface Web, the Dark Web is used not only by organisations and journalists, but also by other individuals who wish to exercise their freedom of expression anonymously.
To be able to attribute texts on the Dark Web to authors, Britta Sennewald experimented with the use of various artificial intelligence methods, such as machine learning algorithms and natural language processing.
Gathering data from the dark web is no easy task
Britta had to go through a fair amount of a cat and mouse game of trying to crawl and extract that data, as it is the dark web" says Dr. Kent, who supervises Sennewald. "When they detect you trying to copy and obtain all that data, they try different things to stop you from doing it. So effectively, because of this, she had to develop some good techniques to collect that data."
Sennewald looked at four categories to examine the data: Word for word text, author's writing style, timing of the post and a language model that analyses the text using different algorithms. She used a classifier that worked best for each category and a final voting classifier to combine the results.
"We were able to correctly attribute a post to its author with an accuracy of 80% and to attribute a text to at least the three most likely authors with a probability of 94%," Sennewald reports. "Therefore, authorship attribution is a risk to anonymity on the dark web."
Sennewald's contributions have opened up possibilities for future research exploring different forms of the Dark Web, thanks to the unique data techniques she has developed.
The junior researcher herself was surprised by her success: "This was my very first paper and I'd like to say thank you for this chance to publish it," said Sennewald. "It's pretty amazing that I won. This award is just unbelievable."
The project was funded by the Natural Sciences and Engineering Research Council of Canada (NSERC) and the German Academic Exchange Service (DAAD).
The news originally appeared on the UNB homepage. Author: Kesang Deker.
Editor for H-BRS: Miriam Lüdtke-Handjery